Publication of GPSAR-Results for Training and Test of AI-based Detectors
- Armin Koppert
- Dec 21, 2023
- 3 min read
December 2023 | GPSAR + AI-based Detectors
In the last few weeks FindMine gGmbH was contacted by several institutions and companies starting or planning the use of AI approaches for UAV-based mine detection in the context of humanitarian demining. Some of them asked us for sample data of our UAV-based ground penetrating synthetic aperture radar (GPSAR). So, we have prepared some training and test data out of our measurements for this purpose.
In this newsletter, we publish this training data under the CC-BY-NC-SA 4.0 license, and we would like to invite interested parties to use it for training and testing their algorithms. If you are successful, we would appreciate it if you contacted us for further discussion around your techniques and possible collaborations.
The data we provide is after the basic SAR-processing, which in our case is a back-projection algorithm.
The diagram in Fig. 1 roughly explains the used processing chain. During the GPSAR-flight radar scans are performed, and the received signals are recorded together with the UAV-positions at the times the scans were taken. After the flight the raw radar data and the corresponding localization data are available for processing. The illuminated area to be evaluated is derived from the localization data and defines the integration space of the back-projection. The back-projection coherently integrates the phase corrected energies from all radar scans for each voxel of the evaluated area. By assuming soil properties and using refraction models, the back-projection can account for ground penetration at different depths. The result is a 3D matrix of complex values representing radar reflection intensity over the volume of the evaluated area. The air-to-soil boundary surface is one layer of the matrix.
The data block, which we call reflection intensity matrix in Fig. 1, is then searched for clusters of high intensity values which are likely to be caused by man-made-objects. The man-made objects in the test data are dummies for explosives buried in the ground.
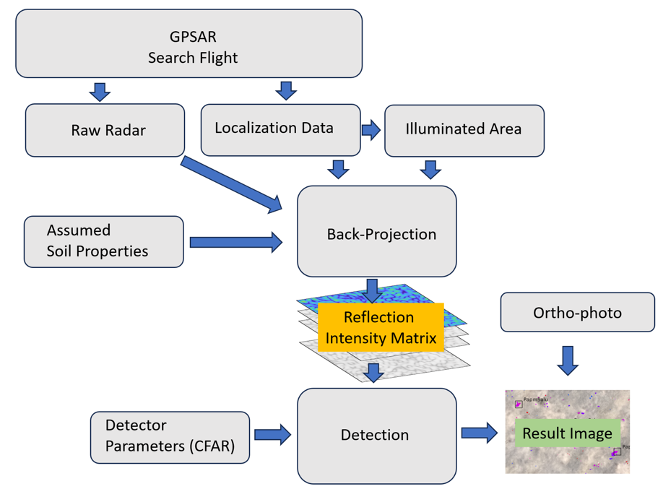
Fig. 1: Simplified GPSAR processing schema.
In accordance with conventional radar signal-processing we call this search-step detection.
In our opinion, the reflection intensity matrix is a reasonable and good entrance point for modern clustering or machine learning methods in our processing scheme. As data is arranged in a rectangular equidistant matrix, it is very similar to general tomographic imaging problems. And there is no necessity for special radar processing as this has been performed in the preceding steps.
We are providing the ground truth of all objects buried in the data sets, to enable both training and testing. In January 2024 we are planning to publish a further data set without ground truth of the buried objects for the case, that someone likes to do a blind test with a trained detection procedure.
The currently used detection algorithm is a simple 2D CFAR. Even with this, we are getting good results in many cases, especially if the soil is dry and vegetation is not too complex. So, our hope is, that there is some improvement with modern AI-based methods.
The training data can be downloaded from our data server and is licensed under the Creative Commons Attribution Non Commercial Share Alike 4.0 International.
The following table gives an overview of the available data sets:
Name/Link | Soil | Area | Depth | Labeled objects |
Sand | 9.9 m x 8.30 m | -0.4 m to 0.4 m | 16 | |
Sand | 9.9 m x 8.30 m | -0.4 m to 0.4 m | 16 | |
Sand | 9.9 m x 8.30 m | -0.4 m to 0.4 m | 16 | |
Sand | 9.9 m x 8.30 m | -0.4 m to 0.4 m | 16 | |
Gravel | 9.4 m x 8.35 m | -0.4 m to 0.4 m | 16 | |
Gravel | 9.4 m x 8.35 m | -0.4 m to 0.4 m | 16 | |
Gravel | 9.4 m x 8.35 m | -0.4 m to 0.4 m | 16 | |
Gravel | 9.4 m x 8.35 m | -0.4 m to 0.4 m | 16 |
Fig. 2 (a-d) give some information on the buried objects.
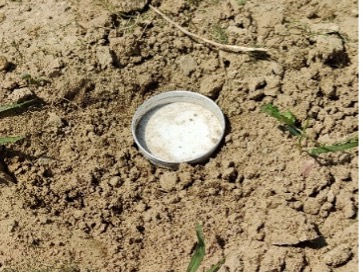
Fig. 2a: Idealized APM-dummy for GPR. Aluminum can lid with diameter of 7.1cm. Stronger and more omnidirectional reflection than Aluminum cylinder of Fig. 2b.

Fig. 2b: Anti personal mine (APM) dummy: Aluminium cylinder diameter 84mm, height 30mm, buried in 5cm depth referred to the top.
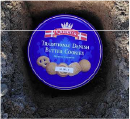
Fig. 2c: Anti vehicular mine (AVM) dummy: Cookie can, diameter 18,7cm, height 10cm, buried in 20cm depth referred to the top.

Fig. 2d: IDE dummy without metal contents. Plastic cylinder half-filled with water.
Details of the format of the provided reflection intensity matrix in the data sets are given in associated readme files and in Fig. 3.

Fig. 3 Description of the reflection intensity matrix. We are using a digital elevation model (DEM) of the air-to-soil-interface and the depth coordinate is relative to the air-to-soil interface. So, the equal-depth areas are following the DEM and for uneven soil, the constant depth areas are uneven, as well.

FindMine gGmbH
Josef-Henle-Straße 3
89257 Illertissen
Germany
Tel: +49 7303 163990
Contact for questions and ideas on findmine
R&D activities, results, technological cooperation.
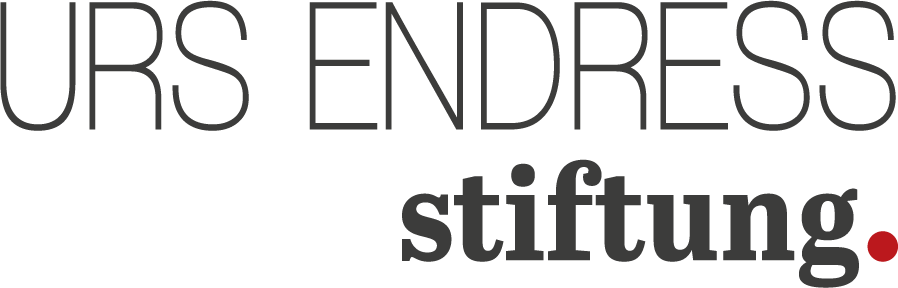
Urs Endress Foundation
Rebgasse 46
4144 Arlesheim,
Switzerland
Contact for general questions on mine action,
and if you are interested to support.